This article has been reviewed according to Science X's editorial process and policies. Editors have highlighted the following attributes while ensuring the content's credibility:
fact-checked
peer-reviewed publication
trusted source
proofread
Computational solution to model 3D RNA structures could speed medical discoveries by decades
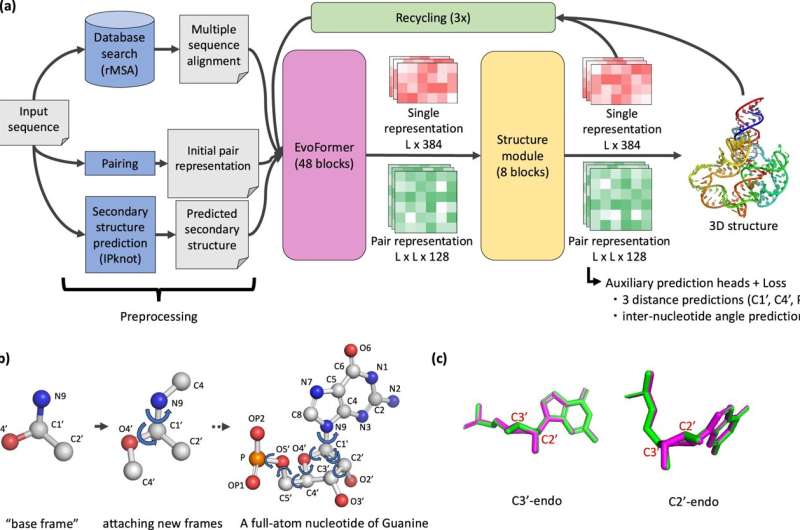
Ribonucleic acid, commonly known as RNA, is involved in many biological functions, and some, including gene silencing, are utilized to cure diseases. RNA has recently gained attention as a promising drug target. Unfortunately, only a small fraction of RNA structures have been determined experimentally, and the process of uncovering these structures requires significant time and effort. Using this time scale, the structures of many lifesaving RNA structures may not be discovered for years. As a result, there is a significant gap between the types of known RNA and the available structural data.
Researchers at Purdue University have developed a computational solution to this problem named NuFold, which will model 3D RNA structures that could expedite medical discovery decades ahead of schedule. The research team, led by Daisuke Kihara, professor of biological sciences and computer science in the Purdue College of Science, has published their findings in Nature Communications.
This result has wide-ranging potential applications, such as in understanding RNA mechanisms and in drug development for diseases involving RNA. NuFold's code and Google Colab notebook are publicly available, making it accessible not only to researchers but also to anyone interested in RNA structural models. NuFold is a groundbreaking tool for more accurate and efficient RNA structure prediction, with the potential to significantly advance RNA research.
In order to sustain life, varieties of molecules must work together. Proteins in these molecules have typically been the primary focus of research, but recently RNA has been found to play unique and broad roles in essential life functions. To understand how RNA functions, it is essential for scientists to know its 3D structure.
Kihara studies protein tertiary structure prediction/comparison, protein-protein docking, protein-ligand docking, protein function prediction, protein sequence analysis, and metabolic/regulatory pathway analysis in the Structural Biology Group within the Department of Biological Sciences. His team is affiliated with the Purdue Institute for Cancer Research and also works closely with the Rosen Center for Advanced Computing (RCAC).
"NuFold is the RNA equivalent of AlphaFold. AlphaFold is the computational protein structure prediction method that got the Nobel Prize in chemistry in 2024," said Kihara. "AlphaFold is now routinely used in many biology labs. NuFold would be an RNA version of AlphaFold. So, in a way, we're extending the breakthrough of AlphaFold into the world of RNA, which has been much more challenging to tackle. By modeling RNA's 3D structure, we can help bridge the gap created by the lack of experimentally determined structures, advancing research on RNA and its crucial roles in life and health."
"It took over three years to develop NuFold," said Yuki Kagaya, main developer of NuFold and postdoctoral research assistant at Purdue. "A key feature of NuFold is how it represents RNA internally, considering the base pairs that are pivotal to the structure while accurately capturing RNA's inherent flexibility. In benchmark tests, NuFold has outperformed traditional energy-based methods and shown better accuracy in local structure prediction compared to recent deep learning-based approaches."
NuFold will help researchers "visualize" how RNA structure would look and boost research progress and drug development with RNA.
"To solve problems that cannot be immediately addressed through experiments, we developed NuFold as a computational solution," said Kihara. "NuFold can help bridge the gap by predicting the 3D structures of RNA from its sequence. By leveraging state-of-the-art machine learning techniques, NuFold can predict the full atomic structure of RNA given its sequence."
The research authors are all affiliated with Purdue. Kihara is the lead author who conceived of and directed the study. Other authors include Kagaya, Zicong Zhang (graduate student, computer science), Nabil Ibtehaz (graduate student,computer science), Xiao Wang (former graduate student, computer science), Tsukasa Nakamura (postdoc, biological sciences) and Pranav Deep Punuru (undergraduate student, biological sciences). Kagaya is the main developer, and the rest of the team participated in coding and benchmarking and developing the Google Colab web server.
More information: Yuki Kagaya et al, NuFold: end-to-end approach for RNA tertiary structure prediction with flexible nucleobase center representation, Nature Communications (2025). DOI: 10.1038/s41467-025-56261-7
Journal information: Nature Communications
Provided by Purdue University